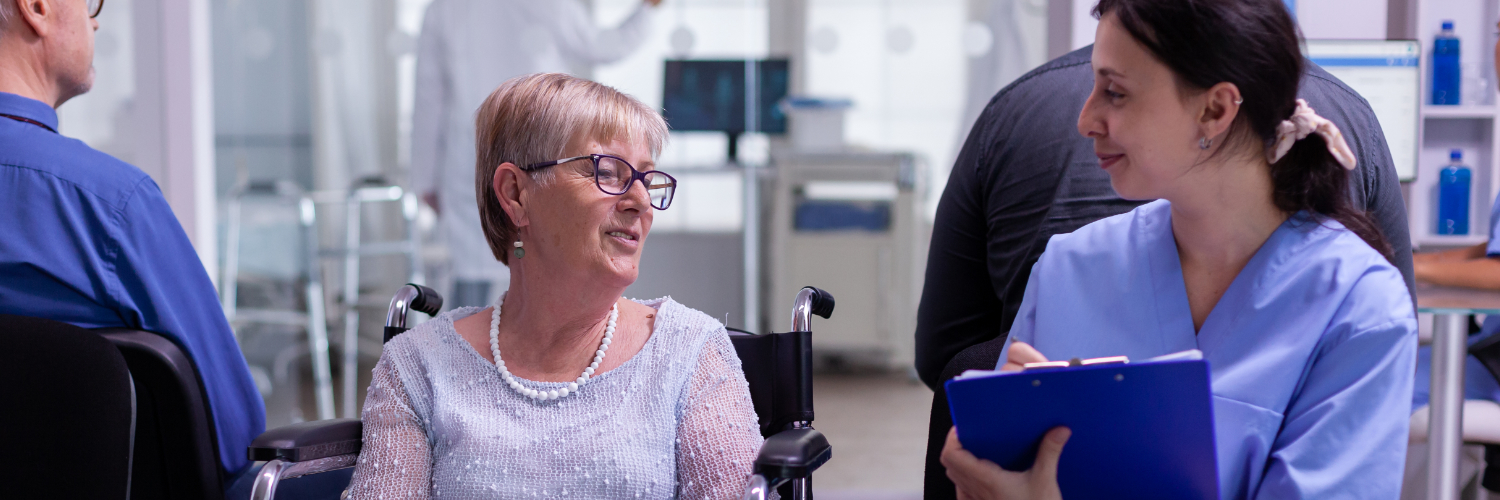
Off-Course to Tip-Off. Automatically Detecting High Risk Patients using Real-Time Patient Record Data
Mission Area
Healthcare
Problem
- We have all seen the headlines over the winter period, elderly and frail patients unable to get a bed and often crammed in ward hallways.
- What is less reported is the often devastating situation of not being able to get back out again.
- This issue effects not just elderly, frail or complex cases, but can touch any one of us; it can be as simple as the right medication required for discharge not being available, or the slot for a minor operation being bumped, leading to needlessly long stays.
- These stays have been shown to lead to ‘decompensation’, the process of becoming sick again, and leading to a much higher likelihood of serious complications and death.
- The effects are more wide-ranging, as those wasted bed days ripple through waiting lists and accident and emergency departments (EDs), causing delays and poorer outcomes for all patients.
- One third of all beds are blocked, for one reason or another, and on top of the negative health outcomes, this costs NHS England approximately £600M a year.
Solution
We have been working with Gloucestershire Hospitals NHS Foundation Trust, who mirror many large trusts across England; approximately 4% of admissions go on to stay longer than 21 days, taking up 34% of all beds - using 278 beds per day that would otherwise be available. We first used historical data to explore ways of using only the information gathered by clinical staff in ED, to flag which patients would become long-stayers; scoring each patient in ED with a score between 1 (very low risk) to 5 (very high risk).
This approach worked well, identifying around 66% of all long-stayers before they were even admitted to a ward (that is, they got given a score of 4 or 5). But we found that the warning signs evolve through the patient stay: in order to be more accurate, and also provide transparency and accountability to the scoring of high risk patients, we needed to follow the patient through their pathway. So we set about building a system that would not only estimate whether the patient would long-stay, but give an accurate estimated day of discharge to the clinician, based on the patient’s evolving record; so that the hospital could plan effectively to support the discharge of the patient.
The system workflow, providing a risk score, a machine-learning estimated day of discharge, and risk factors for the
patient as their stay progresses.
An Integrated Effort
Our team comprised AI engineers and medical scientists from Polygeist, as well as senior clinicians, nurses, and management from the Trust, and a stakeholder group from the wider Integrated Care Board. Starting with a whiteboard and workshops we worked out who needed the information that would be provided, what key information needed to be presented, how it should be surfaced, and when during the pathway should clinicians interact with it. We pitched clinician scoring against a purpose-built AI, and then looked at the risk factors that were calculated; looking to explore and explain what the AI had found, and how we could use it.
Mission Impact
The system that we eventually settled on, which was integrated into the Electronic Patient Record (EPR) system within the hospitals, was able to give a very accurate (within 12 hours on average) estimate of how long each patient would stay; not just accurately identifying long-stayers, but giving critical risk factors to clinicians so that they could plan around the wider system that forms the integrated care system. So accurate was the system on the development data, that the team decided to run a test on discharged patients over a six-month period; their stay would be run through the system, so we could examine how accurate we would have been should the system have been operational. The evidence was staggering: no change, this technology works.
Each of the computed risk bands, showing the length of stay proportions of patients that make up that band. Most
short-stayers have a low score, and most long stayers have a high score.
Next Winter
The team is working hard to move this ever closer to use on clinical wards. Now the real hard work begins, making sure that this technology is correctly used, and as importantly is heeded. We hope that in the coming winters there will be many more beds spare for those that need them, and that automatically identifying high-risk patients will be as routine as automatically reading a pulse or blood pressure.