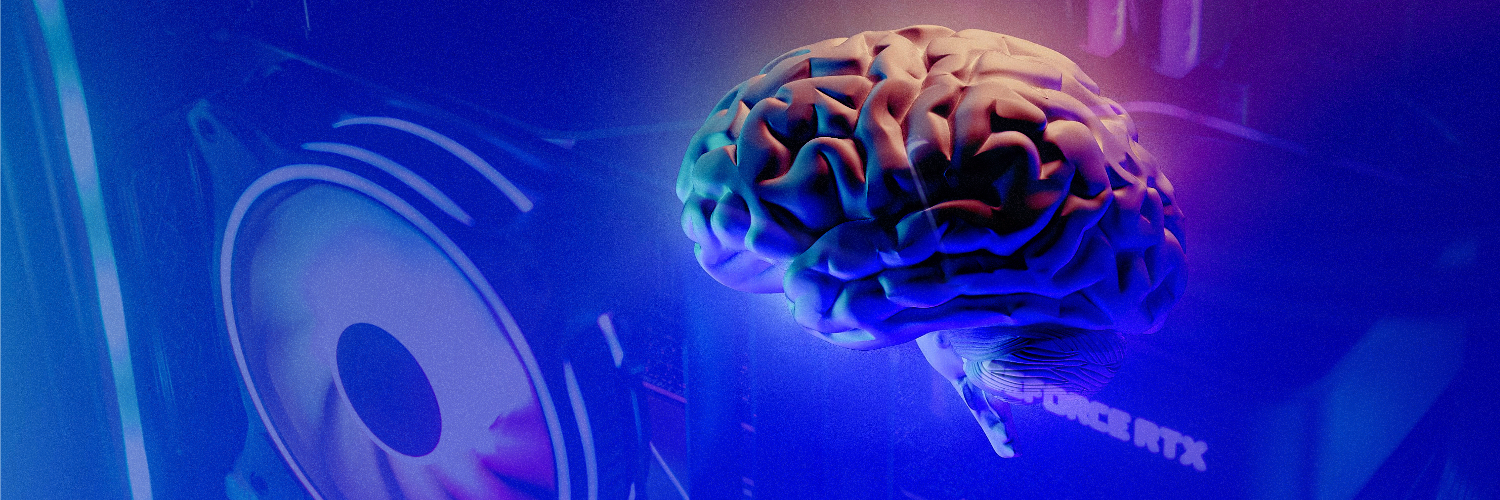
Revolutionising Parkinson’s Disease Detection with a Litmus Test using Deep Learning
Parkinson’s Disease (PD) is a neurodegenerative disorder that affects millions of people worldwide. Advancing the study and treatment of PD means investigating the brains of those who have had PD during their life, and learning what caused those symptoms. However, diagnosing PD from post-mortem brain slices is a time-consuming process that requires highly trained neuropathologists and can take many hours per case. This is a bottleneck in advancing PD pathology research, and slows down the entire research process. However, a recent study conducted by a team of neuroscientists and subject-matter experts from Imperial College London, Parkinson’s UK, and Polygeist has demonstrated a breakthrough in PD detection using deep learning.
This study aimed to develop a tool that could automatically load, enhance and classify brain sections containing alpha-synuclein (α-syn), the pathological marker of PD. The team used a dataset containing digitized images of brain sections from the Parkinson’s UK Brain Bank at Imperial College London. This marker, α-syn, is like finding a needle in a haystack; the haystack being the millions of neuronal fibers in highly magnified brain matter. A thorough and precise measurement of α-syn has never been demonstrated before, due to the process being both very manual, and the shape of the α-syn varying wildly.
The initial goal of the project was to make α-syn more visible to the pathologist by producing a tool that could ‘synthetically stain’ the protein. This goal was quickly achieved, with the tool marking regions of interest in high-contrast bright green. Statistical analysis showed that very few regions in the control group were stained compared to the PD group, suggesting that an automatic classifier could be developed on the basis of this synthetic staining protocol.
The team designed a bespoke neural network model that processed the synthetically stained segments of each immunostained section and produced a binary judgment on whether a segment contains PD pathology or not. The model achieved over 90% sensitivity for PD detection, much higher than the reported sensitivity for neuropathologists. What’s more, the bright green staining could be easily quantified, allowing a direct measurement of α-syn for the first time. This measurement correlated highly with the staging process, known as Braak staging, a number which identifies the severity of the disease and its progression. Take a look below for an example of the processing pipeline.
Brain slices are turned into small stacks of images, and then synthetically stained to produce identified bodies. These
bodies can then be counted and measured like a Parkinson’s Disease Litmus Test.
The Future of Pathology Scanning
This breakthrough has the potential to revolutionise PD detection by saving time and improving accuracy. The use of deep learning in medical diagnosis is an exciting development that could have far-reaching implications for the future of healthcare. For example, this type of approach could be applied to biopsies of living tissues in other pathologies. The team at Polygeist are working on a software library that will be made available to allow other researchers to use this technology, in the laboratory and on hardware such as microscopes. If you would like to know more about this technology, book a briefing with the team.